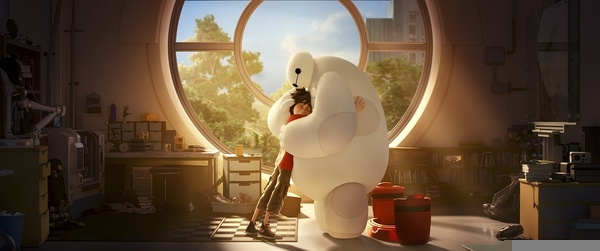
[In] this article is an excerpt from the public accounts "machines of the heart" (almosthuman2014), the English original the Spetrum of IEEE, writer Lee Gomes, produced by machine-the heart of the exclusive translation. Full-text links.
Lee Gomes of IEEE Spectrum, and LeCun (Facebook Director of the artificial intelligence laboratory, Convolutional neural networks) and had a deep conversation, this article excerpt and AI definitions and the most closely related to the several parts.
8 words to explain "deep learning"
IEEE Spectrum: these days we have seen a lot of news about deep learning. In these many descriptions of deep learning, which one do you dislike most?
Yann LeCun: I don't like the description is "it works like a brain", is the reason I don't like it when people say that, although the biological mechanism of deep learning from life inspiration, but it actually works with the brain differences are very large. Analogies with the brain give it the aura of something magical, this is dangerous. This will cause the hype publicity, we asked some unrealistic things. AI went through several winters before because they require some AI can't give something.
Spectrum: therefore, if you are a reporter for a focus on deep learning, and, like all journalists did, with only eight words to describe it, what would you say?
LeCun: I need to think about it. I think there will be "world machine" (machines that learn to represent the world). Another description is "machine learning from end to end" (end-to-end machine learning). This idea is: a learning machine, every component, every stage can be trained.
Spectrum: your editor might not have liked it.
LeCun: Yes, the public will not be able to understand what I mean. Well, there is another way. You can study as the depth is, by consolidating a lot based on the same training modules and components to build the learning capacity of the machine, such as pattern recognition systems. Therefore, needs a training every single principle of things. But this is more than eight characters. Victorias Secret iPhone 6 Plus Case
Spectrum: which thing is the depth of learning systems can do, and cannot be done by machine learning?
LeCun: that's a good question. Before the system, I think we can call it "superficial learning system", will be limited by complexity of functions they can calculate. Therefore, if you are using a similar to "linear classifiers" of superficial learning algorithms to identify images, you will need to be extracted from the image characteristics to provide enough arguments to it. But manually design a feature extraction is difficult and time consuming.
Or you can use a more flexible system of classifiers, such as "support vector machines", or two-layer neural networks, image pixels directly available to them. And the problem is that it doesn't improve object recognition to any degree of accuracy.
Spectrum: it sounds like a simple explanation. Maybe that's why those reporters will try to portray deep learning ... ...
LeCun: like our brains.
There are 500 million switch black box
Spectrum: one problem is, machine learning is extremely hard to get a non-professional field of study. Some layman can understand some technical problem, for example, Google uses the PageRank algorithm. But I bet the only Professor to the linear classifier knew and vector machines. Essentially, this is because the field is very complicated and difficult to understand?
LeCun: actually, I think that the basic principles of machine learning is very simple and easy to understand. I have explained this to high school teachers and students, a topic, and not many of these people find it boring.
Pattern recognition system as a black box, equipped with a camera on the back, with a red light and a green light on top, ahead with a series of switches. A learning algorithm trying to switch, for example, when a dog appears in the camera control switch lights up when the car appeared on camera control switches enable lights. To train the algorithm, you put a dog in front of the machine, if the red light is on, nothing is done. If blurry, twist the knob to make the lights brighter. If the green light is on, twist the knob to make the lights dimmed; then into cars, twist the knob to darken the Red or green light. Many times if you try and keep each knob gradually trimming and, ultimately, machines can always arrive at a correct answer.
Interestingly it can correctly distinguish it have never seen cars and dogs. Trick is to calculate each time you twist the knob the direction and extent of, rather than move. It contains a "gradient" calculation, each twist of the knob represents the light of change.
Now imagine there is a box with 500 million knobs, 1000 bulbs, trained it with 10 million pictures. This is a typical depth of learning systems.
Spectrum: I think you use "superficial learning" the words seemed not too rigorous; I do not think that those who use a linear classifier will think that their work is "superficial". "Deep learning" there is no media in the presentation? Because it looks like it was learned there, but in reality, the "depth" refers only to the stages of the system?
LeCun: Yes, it is kind of funny, but it reflects the real situation: superficial learning system with one or two layers and deep learning systems generally have 5-20 layers. Shallow depth was not referring to study behavior itself, but is training structure.
Hype look like science, but it is not Victorias Secret iPhone 6 Plus Case
Spectrum: hype certainly is harmful, but why do you say this is "dangerous"?
LeCun: because it's to the Foundation, the general public, potential clients, start-ups and investors had expected, they will believe that we are on the cusp-we are building some powerful system like a brain, but in reality we are far closer to this goal. This can easily lead to another "cycle of winter".
Here will appeared some "Schock family science" (cargo cult science), this is licha·Feiman of expression, refers to description some things seems science, but actually not (Translator Note: this from licha·Feiman 1974 in California Polytechnic College of one graduated ceremony speech, description some things seems science, is missed has "Science of character, is for Science thinking Shi must comply with of honest principles").
Spectrum: can you give some examples?
LeCun: "Schock family science", your machine representation is often copied, but no deeper understanding of the principles behind the machine. Or, in the field of aviation, full replication when you make birds look, its feathers, wings, and so on. 19th century people love to do it, but achievement is very limited.
In the field of artificial intelligence, too, they tried that we know of neurons and synapses copies all of the details, and then started on a super computer simulation of a large neural network, hoping to breed in artificial intelligence. This is the "straw bag science" artificial intelligence. There are many getting large sums of funds to support serious researchers are going to believe this.
Spectrum: do you think IBM's True North project (translator's Note: IBM class brain chip integrates 5.4 billion silicon transistors, 4,096 cores, 1 million "neurons" and 256 million "synapses") belong to the "Schock family science"?
LeCun: it sounds a little harsh. But I do think mark claimed by the IBM team and easily lead to misunderstanding. On the surface, their announcement is impressive, but not actually achieving any worthwhile things. Before the True North, the team used IBM supercomputer to "impersonation a mouse's brain". But this is just a random neural network, in addition to consuming CPU operation cycle did not play any role.
True North chip's tragedy is that it could be useful, if it didn't stick with biology to come too close and did not use "spiking integrate-and-fireneurons" model. So it seems to me--I was a chip designer prior to--when you're working on a chip, you must make sure that there is no doubt it can do some useful things. If you create a Convolutional network chips – very well how to do it--it can be immediately used in computing devices. IBM created the wrong things, things that we cannot use it to accomplish anything useful.
Spectrum: there are other examples?
LeCun: fundamentally, the EU plan of the human brain (Human Brain Project) are mostly based on the idea that: we should build a chip simulation of neuron function, the closer the better, used to build supercomputers and chips, when we open it with some learning rules to it, artificial intelligence emerged. I know this is nonsense.
Yes, I said earlier that the EU plan of the human brain. Everyone involved in this project is not satire. Many people involved in this project simply because it can get heavy government subsidies that they can't refuse.
Unsupervised learn-machine learning
Spectrum: for machine learning in General, and how much is yet to be discovered?
LeCun: too much. We in the actual depth of the learning system using learning styles there is still limited. Play a role in actual practice is "supervised learning". You show a picture and tell it to the system is a vehicle, it will adjust its parameters and in the next say "car". Then you can show it's a Chair, a person. Hundreds of examples, take a few days to a few weeks of time (depending on the system), it is understood.
But humans and animals is not the way to learn. When you were a baby, you're not being told the names of all the objects you see. But you can learn concepts of these objects, you know the world is three dimensional, when I was in another object behind you still know it's there. These concepts are not born that way are you learned them. We call this type of study is called "unsupervised" learning.
Middle of 2000s, many of us were involved in the revival movement of deep learning, including Geoff Hinton, Yoshua Bengio, and myself--this is known as the "deep learning groups"-and Andrew Ng, from using non-supervised learning unsupervised learning and philosophy began to rise. Unsupervised learning network can help a specific depth "training". We made a lot of achievements in this area, but can be used in practice or in the past with Convolutional networks combining excellent supervised learning, we were 20 years ago (1980s) thing to do.
From a research point of view, we have been interested in unsupervised learning how to do. We now have a practical technology of unsupervised, but the problem is, we only need to collect more data, coupled with supervised learning can beat it. That is why at this stage of the industry, the application deep learning is basically a supervised. But the future is not going to be that way.
Essentially, in terms of unsupervised learning, the brain is far better than our model, this means that our artificial intelligence learning systems lack many of the basic principles of the biological mechanism of learning.
Old "Singularity Theory"
Spectrum: you said before, do not agree that the "the singularity movement" point of view. I'm interested in is how you look at the social problems associated with it? Its so popular in Silicon Valley, how do you interpret this?
LeCun: it's hard to tell. For this I am a little confused. As Neil Gershenfeld (translator's note, The Director of the Center for Bits and Atoms at MIT) pointed out that the initial portion of the sigmoid function is the exponential rise, it also means that now looks like index growth trend is likely to be bottlenecks in the future, including the physical, economic, and social aspects, followed by experienced inflection point, and saturation. I'm an optimist, but it is also a realist.
Sure some will preach the Singularity Theory, such as Ray Kurzweil. He is a classic Futurist, future empirical point of view. Large handful of singularity, he sold a lot of books. But as far as I know, he was artificial intelligence making any contribution. He sold a lot of products, some of which have a certain degree of innovation, there is no conceptual breakthrough innovations. Sure he didn't write any guide how people in artificial intelligence breakthrough and progress papers.
Spectrum: do you think he has what in Google's seat?
LeCun: so far, it seems few.
Spectrum: I also noticed that when I talk to some researchers the Singularity Theory, there is a very interesting phenomenon. Privately they like this (the singularity) very much, but to the public, their comments will moderate a lot. This is because all the big Silicon Valley believe that reason?
LeCun: artificial intelligence researchers need to ensure that a delicate balance: the goal to remain optimistic, but not to oversell. Needs pointing out is not easy, but cannot make people feel hopeless. You need to be honest with your investors, sponsors, and employees; need to be honest with your colleagues, peers; also needed outside the public and be honest with yourself. When future progress when there is a lot of uncertainty, especially when those dishonest and self-delusion is always about the future success of bombast, this (to remain honest) is difficult. This is the reason why we didn't like unrealistic hype, this was done by people who are dishonest or lying out, but will make those rigorous and honest scientists work more difficult.
If you are Larry Page, Sergey Brin, and Elon Musk, and Mark Zuckerberg's position, you have time to think about the long term technology direction. Because you have a lot of resources, and can make use of these resources for the future in the direction you think better. Inevitably you have to ask yourself these questions: in 10, 20 or even 30 years later, what technology will be like? The development of artificial intelligence, singularity, and how ethical issues will be?
Spectrum: Yes, that's right. But you have a very clear judgment about how computer technology development, I do not think you would believe that we can achieve over the next 30 years to download our consciousness.
LeCun: not anytime soon.
Spectrum: perhaps never.
LeCun: no, you can't say never. Technology accelerates, with each passing day. Some of the issues that we need to now begin to pay attention; while others are distant, perhaps we can point in the science fiction text, but there is no need to worry.

1217 votes
Thunderbolt money treasure
Is designed to help users share money treasure is a little idle bandwidth box, place it at home on the router, you can use to make money. Itself does not have the router function, can be considered a micro server, users insert a storage device such as USB flash drive or an external hard drive, you can make this server a thunderbolt using idle bandwidth to the server part of the network. Contribution to Thunder Server network, the greater, it means digging "mines" more. Dig the minerals can be bound directly to the public, "Crystal mine" convertible into cash red packet, used to spend or bank card.
View details of the voting >>
No comments:
Post a Comment